AI for Healthcare: A Guide for Behavioral Health Providers
Look beyond the hype of artificial intelligence and discover the most vital facts you need to know about AI for healthcare in a behavioral health setting.
Read the guide below or enter your email to download your copy to take on the go.
Introduction
In a recent poll by the Medical Group Management Association, 80% of medical group leaders said they believe the use of artificial intelligence (AI) will be an essential skill, with some respondents indicating they felt it already is. While there remains some measure of caution around concerns like security, ease of use, and excess hype of AI tools, the overall outlook seems positive, with respondents identifying innovation and convenience as the top two traits likely to define AI for healthcare in 2024.
Providers of services for behavioral health and intellectual and developmental disabilities (IDD) stand to gain much from advances in AI algorithms and machine learning-backed solutions that can support them in both direct care and operational responsibilities. However, the successful adoption of these technologies still comes with many questions that should be addressed before choosing the right solutions that will be strong fits for your organization. In this guide, we’ll take a deep dive into the AI for healthcare landscape, with a focus on behavioral health IT, and what you need to know to feel confident and prepared as you begin integrating AI tools into your workflows.
The Current State of AI for Healthcare in Behavioral Health
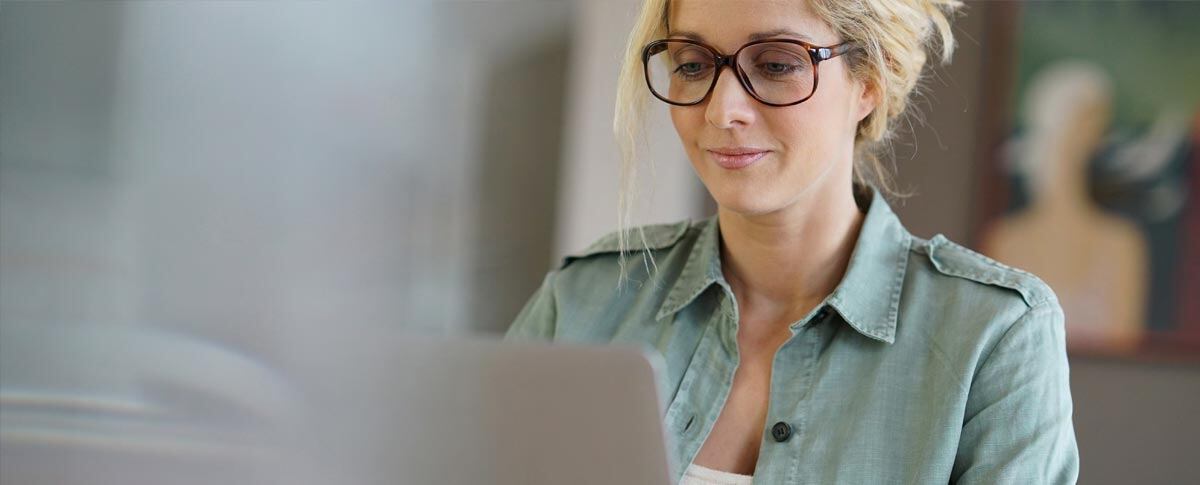
As with any popular new technology, developers and users are keen to see how much artificial intelligence can improve their lives. In many cases, it’s become so ingrained in our routines that we may not even think of its various use cases. From virtual assistants to personalized recommendations to ambient technology, AI is pervasive in our modern world.
A wide array of AI software and services are being rolled out across behavioral health, enhancing the care journey at multiple touchpoints and helping ensure that time-strapped providers are maximizing available resources. In this chapter, we’ll discuss those advantages and how they can help behavioral health leaders maintain their high standards and even improve the provision of safe, secure, and equitable care.
IDD, Substance Use Disorder, and Mental Health AI: Assessing the Benefits
There’s perhaps never been a more important time to bolster the capabilities of providers who care for people with mental health conditions, substance use disorders, and IDD. Over 7 million people in the U.S. have IDD, yet only 19% of that population is receiving long-term support or services. There's also been a 25% rise in mental health cases and a significant increase in substance use disorders since the COVID-19 pandemic.
With AI-powered technologies, providers can better serve existing and new clients with more accurate diagnoses, improvements in how clients experience treatment, and acceleration of workflow efficiencies.
Promoting Pre-Care Productivity
Care for people with behavioral health conditions begins before they even start treatment, with education and emotional support. As those individuals become clients and start meeting with providers, both parties also need tools that help them begin communicating in a productive way.
AI is delivering, with solutions like:
-
Mental health AI tools such as Core Solutions’ ambient dictation technology, which records spoken sessions and summarizes them to reduce provider burden and enable better care
-
Smart chatbots that facilitate scheduling and client-provider conversations or help clients through duress, with the ability to direct them to human providers in emergencies
-
Brain-computer interfaces, which similarly expedite connections between providers and clients with IDD, who may have difficulty expressing their needs
Delivering Better and Earlier Diagnoses
Artificial intelligence also excels at rapidly analyzing massive datasets and deriving insights that can enhance diagnostic practices.
In the IDD population, for example, providers are using AI to analyze facial details, which can help identify disabilities in children. AI algorithms can also detect patterns in children’s language that might signal a condition like autism spectrum disorder (ASD). Mental health AI and machine-learning technologies can record vital signs and other physical data and analyze whether it points to a potential condition. AI is also helping with clinical decision support at the point of care by identifying symptoms and diagnosis trends from notes across the ecosystem.
Further analysis shows not just the power of AI to support highly accurate diagnoses, but also to potentially save lives with its predictive abilities. One clinical team used AI to analyze Facebook and Twitter (now X) posts to successfully predict 79% of clients who were unlikely to complete a treatment plan for a substance use disorder.
Since 85% of people with one of these disorders relapse after one year of treatment, using this kind of data to proactively engage clients can keep them on track and prevent tragedies.
Supporting the Treatment Journey and Beyond
Providers are also gleaning significant benefits from AI in the treatment of clients with substance use disorders or mental health conditions.
Recently, a team of scientists developed an AI-powered wearable that continuously monitors body functions. Clients with substance use disorders can wear the device throughout treatment to relay signals back to their providers, providing a consistent view into their health status and potential drug use.
Artificial intelligence solutions have aided IDD service providers as well:
-
One team that’s aiming to better understand the impact of applied behavior analysis (ABA) therapy on the long-term mental health of children with autism is using machine learning to group and study clients based on how they received ABA. They’re also using AI to create safer medical environments for people with IDD by making imaging machines faster and quieter.
- Some providers are using AI tools — like real-time captioning, sign language translation, and virtual reality — to better engage with and empower clients.
The benefits of AI for behavioral health are clearly numerous. But convincing staff and providers of its value for their day-to-day work may still take some effort.
Is Using AI Technology in Healthcare Okay for Behavioral Health?
Pivotal technological innovations like electronic health records (EHRs) and telemedicine have revolutionized the healthcare industry. However, new technologies like artificial intelligence also inherently come with questions about their benefits and risks. This uncertainty can drive provider discomfort and pushback and ultimately stall adoption.
It’s natural for providers — including those specializing in behavioral health — to express hesitancy around AI technology in healthcare if they’re not familiar with how it’s used. However, organizations are more likely to reap its benefits when leaders stay current on AI best practices and choose vendors that prioritize critical issues for providers.
Here’s a look at the top provider barriers to AI adoption and how to help mitigate them.
Putting Safety First, Last, and Always
Client safety is always top of mind for providers, and AI solutions hold the potential to streamline better and safer care, resulting in improved outcomes. But identifying the right AI technology in healthcare requires understanding how it specifically supports secure treatment, and when more monitoring is required to ensure the AI delivers on its promise.
There have unfortunately been cases of early AI-powered technologies with gaps in algorithmic design or fact-checking. Some text-based therapy AI and cognitive behavioral therapy (CBT) tools have shared content promoting eating disorders to 23% of user prompts. Other tools have “hallucinated” facts without verification. These falsities can impede treatment or recovery.
The good news: There’s more sophisticated AI technology in healthcare, like Core’s tools that support backend processes for behavioral health providers. Core’s diagnosis tracking tools scan provider notes to surface difficult-to-see symptoms and patterns, and its evidence-based practice (EBP) solution supplies guidance for treatment plans ideally suited to each client. These technologies not only boost the safety and quality of care, but they also simplify complex processes, enabling providers to spend more time doing what they do best: caring for clients.
The Data Security and Privacy Question
For behavioral health providers, securing sensitive client data is not only a legal requirement but also an ethical obligation. However, providers may be unclear about whether and how AI technology in healthcare will store, use, and circulate that data.
It’s therefore crucial to understand Health Insurance Portability and Accountability Act (HIPAA) compliance, providers' responsibility in safeguarding protected health information (PHI), and whether the AI in use securely transmits data to the internet.
Behavioral health leaders must have transparency into AI technology vendors’ data security practices. If, for example, a vendor shares information with entities not covered by HIPAA, it's possible that those entities will share client data that’s not anonymized according to HIPAA standards, and that information will then be “re-identified” when combined with other data.
When integrating new AI tools, it’s also the perfect time to upgrade your organization’s security measures with better encryption, identity verification, access controls, and incident response and disaster recovery plans.
Committing to Ethical, Client-Centric Care
For all the convenience artificial intelligence offers, healthcare consumers still value face-to-face time with providers. According to one survey, 63% of people are concerned that AI tools will lead to less of this direct interaction.
The biggest concern that consumers and providers have with overreliance on AI is algorithmic bias, or the cultural biases that developers and users have that can get embedded into the technology itself, potentially informing unfair clinical decision-making.
There are three categories of bias:
-
Illegal bias, or models that break the law, like discriminating against social groups
-
Unfair bias, in which unethical behavior like favoring one gender or political viewpoint over another is embedded in the model
- Inherent bias, which is tied to data patterns that machine learning systems are projected to identify
The first step to combatting bias in AI technology in healthcare and keeping it out of your organization is to ask vendors how they mitigate that bias in their products.
One study recommends AI solution developers evaluate the end-to-end development process to help ensure equity across the product lifecycle. Other sources suggest regularly auditing technologies to keep them aligned with evolving updates.
At the point of care, organizations must also use diverse sources of information to ensure fairness — including surveying clients on social determinants of health (SDOH) — and monitor the inputting of data into the platforms they use.
Of course, AI is still evolving, and it’s critical to maintain awareness of potential bias at all stages of care. Providers must remember to carefully interpret AI outputs and outline processes to catch and fix errors.
Is it okay to use AI technology in healthcare? It is when staff and clinicians understand how to use it securely to supplement their roles and when the companies developing those solutions make safety and fairness a top priority.
Bias and Equity in AI for Mental Health, IDD, and Substance Use Disorders
Behavioral health providers have been diligently working to untangle access to care and care delivery from inequities, so the concern about bias in artificial intelligence algorithms and how to eliminate or mitigate it in AI for mental health, substance use disorders, and IDD care is a subject that warrants deeper discussion.
As these tools make their way into organizations and care workflows, providers must understand the possible impact of AI on underserved populations, follow best practices for adopting fair and equitable AI, research how vendors are tackling bias in the AI solutions they offer, and choose partners that prioritize solutions free from potential discrimination.
The Importance of Addressing Bias in AI for Behavioral Health
These steps are imperative because despite more public understanding of mental health disorders in recent years, receiving treatment for behavioral health conditions still frequently carries stigmas, especially in marginalized communities where medical research, diagnoses, and practices have historically been influenced by cultural biases and prejudices. These systemic problems foster unequal conditions that can significantly impact individuals’ access to care and well-being.
Consider the following:
-
Although 18% of the general U.S. population with substance use disorders get treatment, only 10% of Black, 8% of Latinx, and 3.5% of Native American people do.
- Queer individuals, women, and other marginalized genders have higher rates of substance use disorders and mental health conditions, but they face serious barriers to support.
Behavioral health providers are responsible for addressing these inequities and preventing further harm caused by unintentional AI bias. This is crucial for improving access to care and treatment in racially diverse populations. Data shows that Black people experiencing psychological disorders are incarcerated more frequently than individuals of other races. And when clients of color with IDD receive substandard care in geographical areas with high rates of ableism and racism, their quality of life meaningfully decreases.
AI’s Role in Equitable Healthcare
AI for mental health and substance use disorders may actually, in some cases, be the key to eradicating inequitable treatment — but not before providers first address its shortcomings.
AI’s reliance on human-provided data to train its algorithms and the vulnerability of those algorithms to human biases can not only lead to the possibility of discriminatory care and treatment but also exacerbate inherent biases. Numerous studies have investigated this issue, including one that found prescriptive AI recommendations influenced providers’ likelihood of seeking police help in mental health emergencies involving Black and Muslim men.
Fortunately, technology and healthcare leaders are guiding organizations on their journey to successfully implement AI and attain better health outcomes for all.
The Bioinfo4women (B4W) program at the Barcelona Supercomputing Center, for example, has shared recommendations to surmount AI biases. And advocates are leading the call for a “fair-aware” approach to AI for mental health, aimed at highlighting equity-focused values and methodologies for integration into AI counseling and clinical support.
Mitigating Bias to Realize the Benefits of AI in Healthcare
Beyond following industry and vertical-agnostic guidelines, providers should also begin assessing their AI readiness, including their current approach to equity and implicit bias, and what questions to ask vendors before investing in solutions.
Transparency is essential, so decision-makers should take their time when scrutinizing their options — exploring subjects like the:
-
Intended purpose behind an AI tool and how it’s used
-
Vendor’s approach to identifying and addressing bias and fairness across all development stages
-
Origins of the data the AI solution uses, including whether it reflects the client population
- Vendor’s alignment with the “AI Bill of Rights”
Securing the right AI platform can help behavioral health leaders gain the ability to foster more trust with both clients and providers and deliver quality care that produces more consistent, positive outcomes. As a final step to maintain that trust, leaders should also ensure they create internal plans to prevent and mitigate bias when and/or if it happens.
How AI for Healthcare Can Advance Behavioral Health
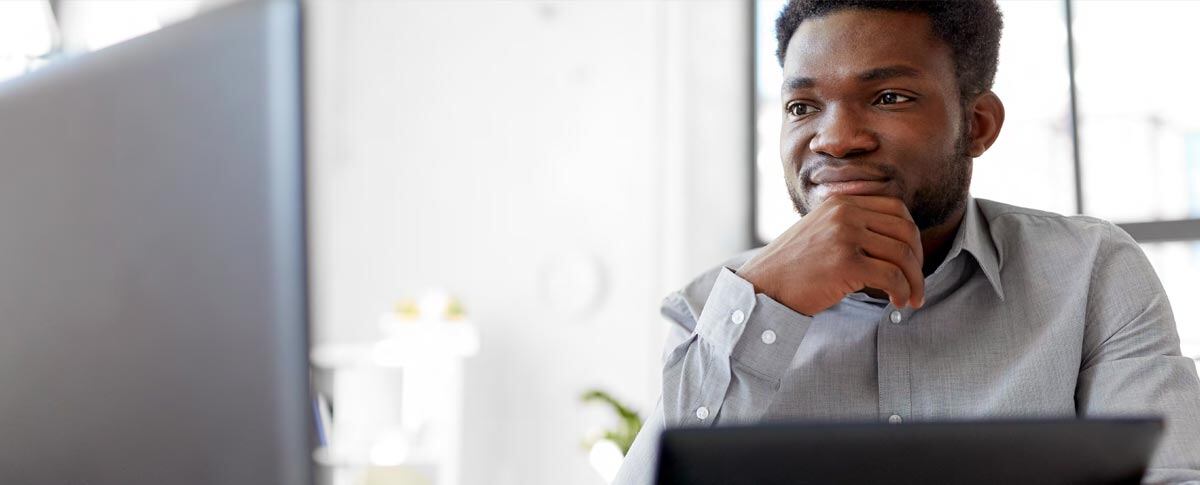
Facilities that thoughtfully prepare for the safe, secure, and fair use of AI in behavioral health put themselves in position to provide cutting-edge care to clients and give clinicians and staff much-needed breathing room as they balance many responsibilities. But AI for healthcare also has impressive potential to impact these fields in even broader ways, increasing the percentage of people who opt in to care by helping break down barriers and improving access to treatment. AI can also bring to light patterns in data and potential long-term dangers for at-risk clients that providers can use to keep them committed to treatment plans.
Healthcare and AI: Overcoming Stigmas in Behavioral Health
Compared to other areas of healthcare, clients receiving treatment for mental health, substance use disorders, or IDD are prone to some of the most persistent stigmatization. Social taboos still prevent millions from pursuing or receiving essential care.
But AI is increasingly offering opportunities to counter this stigma. Let’s preview the future of behavioral healthcare and AI, and how the latter is helping reduce the stigma many individuals feel.
The State of Stigma in Healthcare
The U.S. seems to have recently reached a tipping point for psychological and emotional wellness. A 2022 poll showed that 90% of U.S. adults considered the country to be in a mental health crisis. Drug overdoses skyrocketed fivefold from 2002 to 2022, shockingly exceeding 105,000 overdoses. And in 2023, ECRI deemed the pediatric mental health crisis its most urgent patient safety threat.
Yet, despite behavioral health conditions being unequivocally normal, stigma around them persists, with The Lancet calling mental health stigma a “health crisis.”
Stigmatization hinders individuals’ ability to receive high-quality care. In one study, for example, users of injected drugs often felt dehumanized and faced discrimination in healthcare settings. This led about 10% of people with substance use disorders to avoid pursuing care in 2021. People with IDD — who frequently face social isolation due to stigma — also have lower rates of preventative screening.
For things to change, behavioral healthcare providers need to leverage a growing number of tools at their disposal.
AI Use Cases in Healthcare
To overcome stigma, providers must support individual empowerment and improve individual agency by communicating openly with current and potential clients. This will help ensure clients feel seen and empower providers to better engage clients in their care journeys and provide encouragement when the effects of stigma make sharing information feel difficult or impossible.
SDOH Tracking, Chatbots, AI Mental Health Apps, and Peer Support
Several types of healthcare and AI technology support and supplement these efforts by prioritizing personalized experiences, ranging from tools that improve population health management to apps that expand care and cultivate community outside of facility walls.
Examples include:
-
Core Clinician Assist: SDOH Tracking, Core Solutions’ AI-powered tool that makes information about social determinants of health available to providers at the point of care. This enables providers to better understand the environmental factors influencing a client’s physical and mental health and paves the way for discussions that destigmatize issues like poverty or low-quality education.
-
AI mental health apps and chatbots that people can use to learn about disorders in private, judgment-free settings; dispel myths; and explore treatment paths.
- Peer support-focused AI solutions, which can use AI algorithms to forge connections between people in similar situations, such as those with substance use disorders.
Machine Learning, Healthcare, and AI Algorithms
Even after receiving care, mental health, substance use disorder, and IDD clients may continue to face stigma that thwarts recovery. Healthcare and AI technologies with predictive capabilities, such as machine learning (ML)-backed solutions, can help. ML has the power to not only identify patterns in immense datasets that it reviews in little time, but it can also foresee behaviors, condition progression, and outcomes, and screen for conditions that carry stigma.
These are critical advantages for behavioral health providers who often face client reluctance to truthfully answer questions about their symptoms. ML findings can inform more proactive and tailored care and treatment plans.
The number of ML use cases in behavioral healthcare is increasing. Consider these recent examples:
-
ML helped one team of researchers analyze the health records of over 5,000 clients with mental health conditions. The algorithm had an 84% success rate at predicting whether a client would attempt self-harm within a week.
-
Another ML program examined speech patterns in clients with schizophrenia, and successfully predicted when psychosis would begin 83% of the time.
- One ML program even predicted severe psychological distress better than a team of psychiatrists.
Finding the Right AI for Reducing Stigma
Providers researching their AI tool options should understand whether the technology is designed to help reduce stigmas and ensure more people get the care they need.
Consider asking these questions when meeting with vendors:
-
Has the algorithm been rigorously tested for bias and fairness to prevent discrimination?
-
How does the platform address confidentiality and data privacy?
- Does the AI properly handle communication and language differences?
Machine Learning and Healthcare: Advances in Behavioral Health
Many AI solutions backed by machine learning algorithms can enhance behavioral health diagnostics and treatment. With ML and healthcare rapidly evolving, it’s important for providers to understand how these technologies can augment their work.
Exploring the Foundations of ML in Behavioral Health
A review of machine learning and behavioral health found that within this area of healthcare, ML uses three types of learning:
-
Supervised learning, which evaluates data to forecast outcomes.
-
Unsupervised learning, which trains machines with unlabeled data. This model discovers and reports patterns and features in the data.
-
Reinforcement, which teaches the machine to take appropriate action and maximize rewards based on its environment, like a robot choosing the right peg to put in a hole.
Since ML can identify and sometimes act on patterns, it can contribute to better care journeys and population health research.
AI in Mental Health, Substance Use, and IDD Diagnostics
Machine learning-backed solutions have steadily been supporting earlier and more accurate diagnoses, creating opportunities for quicker interventions and timely preventive care — even slowing down disorder progression, reversing it, or even preventing onset.
A 2021 study, for example, used ML to identify variables that strongly predict future substance use disorders. Other AI in mental health tools are helping providers understand characteristics that contribute to accurate diagnosis of conditions like schizophrenia or Fragile X syndrome.
Improving Treatment Quality With Machine Learning
Technologies that use machine learning are also becoming key factors in creating better, more personalized care and treatment plans. For example, Core Clinician Assist: Symptom Tracking monitors an individual’s symptoms over time and associates them with diagnoses. It scans session notes to uncover symptoms that may not be immediately obvious across provider interactions. It then aggregates the symptoms and rolls them up into their associated diagnoses, providing a clear clinical picture to the provider at the point of care and leading to more tailored behavioral healthcare.
Other use cases for machine learning and healthcare AI include:
-
Pairing an individual with the right therapist by analyzing the person’s mental health needs via a questionnaire
-
Speeding up the imaging experience and making it more comfortable for clients through deep learning — a step forward that is particularly important for helping people with IDD feel safer and calmer in healthcare settings
Artificial Intelligence and Population Health
To step up their efforts to offer equitable care, improve interventions, and allocate resources more fairly, behavioral health providers are examining larger population-based trends by using advanced ML-fueled technologies like Core’s SDOH tracking tool, which is available in the Cx360 platform and as a standalone algorithm accessible via application programming interface (API).
At Georgia Tech, researchers used machine learning techniques to analyze almost 1.5 million Reddit posts from people with substance use disorders and identified alternative treatments people were following to try to recover from opioid addictions and how those treatments were being used. Other studies are utilizing ML and healthcare tools to better understand intellectual disability in Down syndrome and gene mutations associated with developmental disabilities.
Practical Use of AI for Healthcare Across Behavioral Health
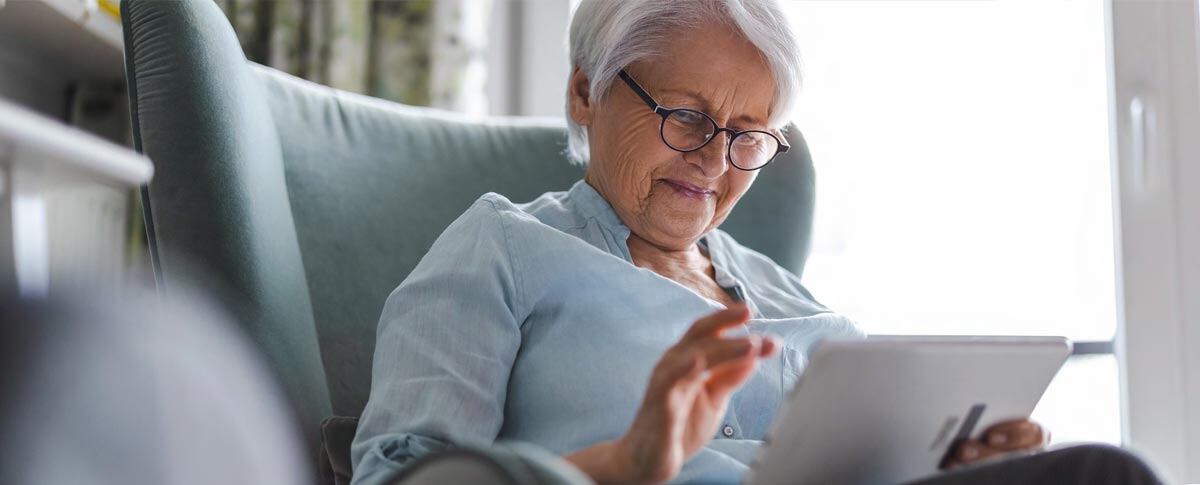
It’s clear that artificial intelligence technologies are being endlessly studied and tested, and research and clinical teams are regularly producing exciting new discoveries and findings on how these AI tools can push behavioral healthcare to new heights. But leaders must be able to win over their teams and make plans for how to implement AI for healthcare services. In this final chapter, we’ll walk through some of the most consequential advantages that AI provides and identify the next steps you should take to choose the most appropriate solutions to meet your needs.
AI Diagnosis Tracking for Mental Health, Substance Use Disorders & IDD
An early and correct diagnosis is the bedrock upon which providers develop successful care plans, but in behavioral health, diagnosing conditions is often complicated. Symptoms are frequently subjective, and clients can struggle to explain them in a straightforward fashion — sometimes making the right diagnosis a moving target.
These challenges are compounded in the primary care and medical arenas by data being entered by a wide array of providers, all at various stages of a client’s care journey. Limited resources don’t allow for a comprehensive review of that information, leaving gaps in behavioral health and SDOH insights.
AI diagnosis and symptom tracking tools can help by analyzing this data and outlining the symptoms clients exhibit. With these insights, providers can diagnose conditions sooner and with greater accuracy.
Diagnostic Difficulties in Behavioral Health
Providers must weigh several complex factors when diagnosing behavioral health disorders, including a client’s sociocultural environment and whether they have co-occurring conditions. But unlike in other healthcare areas, what drives much of a provider’s assessment is client reporting.
The problem is that the subjective nature of an individual’s experience can create an incomplete view of what they’re feeling and the events that motivated them to seek help, so it's unsurprising that misdiagnoses are common. One study reported misdiagnosis rates for major depressive disorder of nearly 66%, and those for bipolar disorder were close to 93%. Another study found that 39% of clients with severe psychiatric disorders were misdiagnosed.
Diagnosing substance use disorders is likewise a manual and subjective process, while IDD diagnostics rely on parent questionnaires, making it difficult to identify symptoms firsthand.
How AI Diagnostics Help Identify Conditions Earlier and Better
With AI diagnosis technology, providers can introduce more objectivity and accuracy into behavioral health diagnostics.
Let’s look at some of the advantages to using AI diagnostics and AI clinical decision support tools.
Earlier Screening
Diagnosing a behavioral health condition early can help a provider better implement an applicable treatment plan and manage symptoms before they worsen.
AI diagnosis solutions evaluate large datasets and identify diagnostic patterns that quickly shed light on symptoms and possible conditions to inform provider decisions. Machine learning solutions are also being used to identify and categorize early signs of addiction.
Better Diagnoses and Decision-Making
AI clinical decision support systems (CDSS) can enhance the efficacy of diagnostics and care regimens by using ML to detect mental health conditions, substance use disorders, and IDD with an 89% accuracy rate, and then facilitating the best clinical decision-making for clients.
Hawaii researchers are using similar technology to diagnose and treat adolescent developmental delays through AI-powered video games.
Personalized Care
No two clients are the same, regardless of whether they have the same symptoms or condition, so it’s vital to customize care to the individual. Artificial intelligence can help here as well.
For example, some researchers used an AI platform to help formulate personalized care and education for individuals with IDD, while others are following people with substance use disorders in an effort to supply tailored coaching and prevent emergencies.
Adopting AI Diagnostics in Your Practice
To determine whether AI diagnosis solutions are right for their organization, providers must first evaluate their current diagnostics, asking questions like: What’s our diagnostic accuracy rate? What disorders are challenging to identify? Which clients get lost in the system when diagnoses are particularly difficult to reach or are inaccurate?
Behavioral health and medical providers may want to consider adding Core Clinician Assist: Symptom Tracking AI. In addition to its uses that we’ve already discussed, such as its ability to scan provider notes at high speed to identify hard-to-find symptoms, the tool has several other benefits. These include behavioral health symptoms trending, intuitive visuals, and, coming soon, diagnosis recommendations aligned with identified symptoms.
Ambient Technology and AI Adoption for Behavioral Health Staff
Artificial intelligence is not only aiding better diagnostics but also speeding and improving service delivery across the healthcare continuum and supporting the attainment of better outcomes. Just as importantly, it’s giving backend operations a big upgrade. With workforce shortages continuing to plague healthcare, tools that can fill in resource gaps and improve productivity are proving increasingly critical.
By streamlining workflows and optimizing administrative procedures, AI solutions such as ambient technology and chatbots are giving behavioral health providers back crucial time they need for working directly with clients and tackling other critical, often time-sensitive tasks.
Behavioral Health AI Solutions for Operational Support
Unmanageable workloads and high client expectations are major contributors to provider burnout, which — according to the Substance Abuse and Mental Health Services Administration (SAMHSA) — has reached rates as high as 78% among psychiatrists. Staff need help with tasks like scheduling and client education to ease operational burdens.
There are multiple examples of AI in behavioral health that significantly drive better operations and outcomes. We’ve already touched on one — AI algorithms — such as the algorithm powering Core Clinician Assist: Symptom Tracking. These algorithms also have other use cases, such as evaluating the behaviors of people with substance use disorders to flag risk of crisis events and track long-term treatment adherence, as well as predicting the likelihood of treatment plan success, which can help guide adjustments. Here are two other key solutions:
Ambient Technology
Many people who use ambient technology may not even know it, as these contextually aware tools work in the background of our daily lives and don’t need much direct user interaction. They leverage artificial intelligence, connectivity, and sometimes sensors to collect data and provide information to users. AI clinical documentation and dictation technologies are one example.
In behavioral health, ambient AI clinical dictation tools like Core Clinician Assist: Documentation record conversations from provider/client sessions and then reformat and summarize the information. Core’s solution goes even deeper, using natural language processing (NLP) to solve a common problem: the lack of a bigger-picture view into a client’s condition. The tool surfaces symptoms from the notes of all the client’s providers to help create a superior treatment plan.
These types of technologies also integrate easily into existing systems and share notes in helpful formats, like the SOAP (subjective, objective, assessment, plan) structure.
Chatbots
Chatbots are ubiquitous in behavioral health, with dozens of use cases from scheduling to communication. They can empower clients to set themselves up for positive care experiences by self-managing appointments, communicating symptoms or concerns that can be escalated to a provider, and reviewing educational resources related to their symptoms, condition, or treatment plan. They're especially beneficial for people with IDD who lack verbal communication abilities or for clients who prefer having options for engaging with providers, using channels like text, video calls, and email.
Similar to AI clinical dictation technologies, these chatbots automate processes and free up provider and staff time — and not just before clients come into a facility. A chatbot for mental health and substance use disorders can also engage clients throughout the recovery process, including contacting them at specific times after they complete treatment. When those individuals type or speak to the chatbot, it can use speech patterns and language choice to identify the potential for relapse.
Core’s upcoming chatbot — in production at the time of publication — will securely integrate into EHRs, enabling providers to verbally navigate them and dictate notes directly into a client’s chart.
Integrating AI in Mental Health, IDD, and Substance Use Disorder Ops
AI is seemingly everywhere in the healthcare ecosystem and has become an increasingly important addition to efforts to improve behavioral healthcare and operations. But implementing AI in mental health, substance use, and IDD services can feel daunting due to the need to address clients’ and providers’ unique circumstances. Time, research, and pointed vendor evaluations are therefore essential to choosing the ideal solutions.
Let's look at five steps you must take on this journey.
1. Take Stock of Your Needs and Challenges
You can’t entrust artificial intelligence with solving your problems if you don’t first understand what those problems are, so building a persuasive case for making an investment should begin with an audit of both the needs of your providers, staff, and clients and the barriers they encounter to having those needs fulfilled.
Gather feedback from operational leaders. Are staff overburdened by excessive work? Are clients unable to easily schedule appointments? Can providers use more assistance to make timely and correct diagnoses?
Leave no stone unturned by looking at:
-
Redundancies in current workflows
-
Gaps in scheduling and care procedures
-
Outdated and inefficient technologies
- Cost structures that don’t drive enough revenue
2. Do Your AI Due Diligence
There are a dizzying number of options for AI in mental health, substance use, or IDD, but not all will be relevant to your needs or worth your investment. Once you’ve captured your organization’s pain points and improvement opportunities, research which specific AI-backed tools are a fit.
Consider what we've reviewed:
-
Ambient dictation. In one survey, over 90% of healthcare providers said their documentation requirements are onerous. Ambient dictation gives providers the ability to record verbal client notes into charts and EHRs, easing this burden and giving them back valuable time for meeting with existing clients or welcoming new ones.
-
Behavioral health chatbots. From automating operations to providing 24/7 virtual support for clients, mental health chatbots offer extensive supplementary support for providers and individuals, and some can alert providers about possible crises.
-
Diagnosis tracking tools. A major impact of AI in healthcare is its ability to enhance diagnostic procedures. AI can analyze massive datasets to identify hard-to-spot symptoms and connect them to possible diagnoses. These tools help providers make more accurate diagnoses earlier in care.
-
AI algorithms. Solutions built specifically for behavioral health specialties — such as AI psychotherapy — can help support operations and care throughout the entire client journey. These solutions can monitor clients’ progress to keep them on track or assess treatment adherence over time.
Core Solutions offers several of these AI-powered technologies in addition to its SDOH Tracking algorithm, and it has solutions to help providers spot anomalies in administrative progress notes and to streamline billing for staff.
3. Put Equity First
Artificial intelligence-powered tools like chatbots and AI psychotherapy aren’t fully effective unless they’re trained on diverse datasets that fairly represent the populations you serve. When human biases are embedded into that data, clinical care decisions are less likely to meet the specific needs of the client and more likely leading to fewer positive outcomes.
The impact of AI in healthcare can be compelling and powerful if you take time to evaluate how and from which sources developers gathered the data the AI was trained on, and what precautions they took to avoid embedded biases. Be sure to avoid AI solutions that indicate illegal, unfair, and/or inherent bias.
4. Prepare for Implementation
Implementing new technology like AI requires change management, so leaders must prepare providers and staff for integration.
Clarify for employees how the AI will impact their roles and care delivery, and train them on:
-
How the solution operates
-
Social and ethical implications of the AI
-
Key steps to evaluate the quality of the AI’s inputs and outputs
-
How to review the AI’s impact on care
If you’re not ready to adopt a full suite of solutions, you may start by integrating just one AI tool into your existing systems and taking it from there. Leaders should bear in mind, however, that leading platforms like Core’s Cx360 can make a more holistic approach feasible while also providing excellent efficiency and many other enterprise-wide benefits. Some of Core's AI tools can be used as standalone add-ons or be integrated with an existing EHR or care management platform via API.
5. Prioritize Data Security, Privacy, and Compliance
If there’s one certainty about security in the healthcare industry, it’s that you must always be prepared to prevent or mitigate data breaches. In fact, in 2023, the industry experienced its highest-ever number of client record breaches, with a whopping 133 million. It's unsurprising, then, that providers and clients are concerned about AI's handling of sensitive data as it circulates through health systems and facilities.
What does this mean? It’s critical that you evaluate an AI technology’s security protocols before committing to a solution. Consider products like Core’s, which have security checkpoints at every development stage and are aligned with the National Institute of Standards and Technology’s standards and best practices.
Choosing the Best AI for Healthcare as a Behavioral Health Leader
It’s easy to get overwhelmed by the many behavioral health AI solutions on the market, but successful implementation of the right tools is achievable when leaders, staff, and providers educate themselves on the benefits of AI for healthcare and the considerations they need to manage to ensure the technology is delivering as intended. When decision-makers dig into the differences between available options and when teams consider the specific needs and problems they face operationally and in the pursuit of better care and treatment, it becomes easier to narrow down the choices of AI tools.
To get the most from your artificial intelligence, take a good look at a comprehensive platform like Core’s, built specifically to meet behavioral health provider- and staff-specific needs. Beyond the Cx360 EHR, which includes telehealth, billing improvements, and workflow management, remember the additional AI-powered tools Core offers, such as:
-
Core Clinician Assist: Documentation, which records sessions with clients to better determine health needs
-
Core Clinician Assist: Symptom Tracking that reviews provider notes to surface difficult-to-find symptoms to improve diagnosis
-
Core Clinician Assist: SDOH Tracking, which creates visibility into social determinants of health at the point of care
-
Core Clinician Assist: Anomaly Detection for flagging possible issues that might affect care
The use of AI in mental health, substance use disorder, and IDD care is rapidly accelerating, and Core Solutions is at the forefront of innovation, with artificial intelligence solutions and a platform that help organizations nationwide elevate their operations, enhance quality of care, and improve outcomes.
To learn what Core can do for you, get a demo today.
Save this information for later.
Download the PDF version now.